The training of Large Language Models has been a challenge. Researchers have been trying to achieve more optimistic and result-oriented ways to make language models that are smaller, cost-effective, and offline. After many efforts, researchers potentially have found the best solution.
Introducing the Microsoft Phi-3 mini, a game-changing advancement in language models trained on 3.3 trillion tokens. This compact marvel, developed by the Microsoft research team, is not only lightweight and cost-effective but also designed with a specific focus on function.
This unique approach represents a significant leap in our comprehension of language processing. The Phi-3 is also a scaled-up version of Phi-2, which was composed of heavily filtered web data and fabricated data or synthetic data.
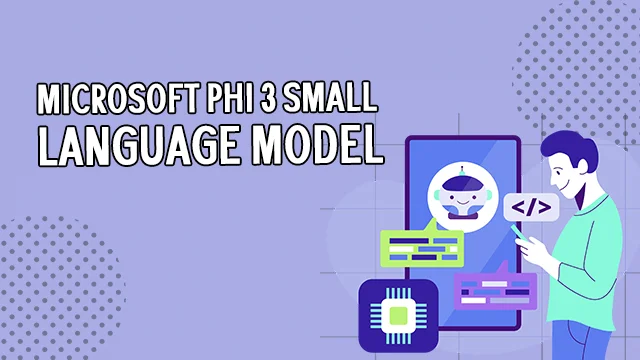
Let’s discuss the following topics in detail.
The Evolution From Large to Small Language Models
A large amount of training data and significant computing resources are required to train large language AI models. For example, to train the most powerful large language model, GPT-4, an estimated $21M was spent in three months of training.
After training in the GPT-4 language model, it becomes powerful enough to perform reasoning functions. But it lacks tiny functions, such as generating sales content or serving as a sales chatbot. It’s like using a flamethrower to light a candle.
Microsoft’s Approach with Phi-3 Mini
The Microsoft Phi-3 family of open models, the most capable SLM, is a compact language model with a significant impact. Unlike its counterparts, the Phi-3 mini is trained over 3.8B parameters, trained on 3.3 trillion tokens, which makes it more powerful than LLMs.
Microsoft claims that this is not only a powerful solution but also an optimal, cost-efficient one for a wide range of functions. The Phi-3 excels at handling various complex tasks, making it applicable in numerous areas.
Phi-3 mini can perform a wide range of tasks, including document summarization, in-depth knowledge retrieval, and content generation for social media. In addition, Microsoft also claims that the standard API of Phi-3 mini is available for developers to deploy in their programs, further expanding its potential applications.
Phi-3 Mini’s Performance Compared to Larger Models on MMLU
Microsoft’s Phi-3 small language models do much better than other language models of similar or bigger sizes on important tests. They ran a test on Retrieval-Augmented Generation, and the results were surprising.
Phi-3-mini models that are still yet to be released perform even better than models that are twice their size.
In addition, Microsoft claims that there are additional models, such as the Phi-3-small and Phi-3-medium, that are launching soon with the Phi-3 family. The Phi-3-small consists of 7 billion parameters, and the Phi-3-medium consists of 14 billion parameters. It does better than much bigger models, like GPT-3.5T.
These two models will soon be available on Microsoft Azure AI Model Catalog, Hugging Face, and Ollama (a lightweight framework for developers to run locally).
The benchmarks evaluate language models, math capabilities, reasoning, language understanding, coding, and other parameters. The numbers determine that the output of the Phi 3 small language models performed significantly better than the large language models.
Microsoft also claims that all the numbers that all the reported numbers are made using the same process to make sure they can be compared.
That means they might be a bit different from other published numbers you’ve seen across benchmarks because of slight differences in how the tests were done. You can find more details about these tests and their overall performance in their technical paper.
Phi-3 Safety Model Catalog
The Phi-3 small language models are developed by Microsoft with a strong focus on responsible AI practices. These models are built in line with the Microsoft Responsible AI Standard, which follows six core principles, such as:
- Accountability
- Transparency
- Fairness
- Reliability
- Safety
- Privacy
- Security
- Inclusiveness
Microsoft has implemented stringent safety measures to ensure the safety and reliability of Phi-3 models. These measures include comprehensive safety evaluations, red-teaming to identify any potential risks, reviewing sensitive uses, and adhering to security guidelines.
These steps are taken to ensure that the development, testing, and deployment of Phi models are done responsibly, according to Microsoft’s standards and best practices.
In addition to these measures, Phi-3 models undergo high-quality training data. They are further enhanced through post-training safety measures, such as reinforcement learning from human feedback (RLHF), automated testing across various harm categories, and manual red-teaming.
Opening the New Horizons Of Capabilities
The Phi-3 AI models developed by Microsoft have various potential applicable areas due to their unique features and capabilities. As the Phi-3 mini initial training consists of small language models, it opens numerous possibilities. Here are some key areas where Phi-3 models can be effectively utilized:
Resource-Constrained Environments
Phi-3 models are well-suited for environments with limited computational resources, including on-device and offline scenarios. Their smaller size allows them to operate efficiently in such settings.
Latency-Sensitive Applications
In scenarios where fast response times are crucial with high data transformation, such as real-time processing or interactive systems- Phi-3 models will provide their services due to their lower latency.
Cost-Conscious Use Cases
Phi-3 small language models also offer a cost-effective solution, particularly for tasks with simpler requirements. Their lower computational needs make it capable of reducing costs without compromising performance.
Compute-Limited Inference
Phi-3-mini, specifically designed for compute-limited environments. That is why it can be deployed on-device and further optimized for cross-platform availability using ONNX Runtime.
Customization and Fine-Tuning
The smaller size of Phi-3 models makes them easier to fine-tune or customize for specific applications, enhancing their adaptability to diverse tasks.
Analytical Tasks
Phi-3-mini demonstrates strong reasoning and logic capabilities. These capabilities make it suitable for analytical tasks that require processing and reasoning over large text content, such as documents, web pages, and code.
Agriculture and Rural Areas
Phi-3 small language model is already proving valuable in sectors like agriculture, where internet access may be limited. They provide farmers with access to powerful AI technologies at reduced costs, improving efficiency and accessibility.
Collaborative Solutions
Organizations, such as ITC, are leveraging Phi-3 models in collaborative projects to enhance efficiency and accuracy. The ITC company developed a copilot farmer-facing software with Microsoft collaboration that aims to improve agricultural practices using Phi-3 for efficient decision-making.
Where To Find Phi-3 Small Language Model?
Those who want to uncover the real potential of this smart technology can visit the Azure AI Playground. In addition, the Phi-3 AI model can also be explored on the Hugging Chat playground. So, make your contribution and use this magnificently powerful and efficient AI model on Azure AI Studio.